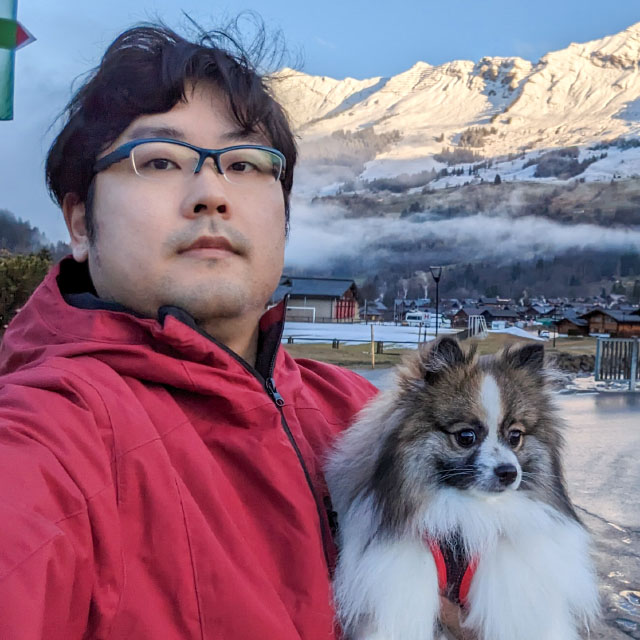
Naoya Takeishi is a researcher working at the University of Tokyo. He is interested in the effective integration of domain knowledge into statistical machine learning and also in data-driven analysis of dynamical systems, whose application ranges from scientific research to industrial machinery.
Contact
ntake[at]g.ecc.u-tokyo.ac.jp
I don’t observe the inbox of old addresses (…@ailab.t.u-tokyo.ac.jp, …@hesge.ch, …@riken.jp).
Emails from the new address (…@g.ecc.u-tokyo.ac.jp) may be marked as spam automatically. If you find no replies from me for a while, please check the spam folder (though it is also likely that I’m just off).
Recent activities
2025-03-01 | Invited talk on hybrid modeling @ the Workshop on Functional Inference and Machine Intelligence [slides] |
---|---|
2024-12-14 | I will be a panelist @ the workshop on Machine Learning and the Physical Sciences, NeurIPS 2024 |
2024-10-22 | Invited talk on ML and scientific models @ The 2024 Fall Meeting of the Seismological Society of Japan [slides] |
2024-09-23 | I joined the Editorial Board of the journal Machine Learning: Science and Technology (MLST) |
2024-07-15 | Invited talk on neural nets and Koopman operator learning @ the workshop on Koopman Operators in Robotics, RSS 2024 [slides] |
2024-05-29 | Tutorial talk on ML and scientific models @ The 38th Annual Conference of the Japanese Society for Artificial Intelligence [slides] |
2023-08-03 | Invited talk on ML and scientific models @ The 46th Annual Meeting of the Japan Neuroscience Society |
2023-07-28 | Co-organized the workshop on Synergy of Scientific and ML Modeling @ ICML 2023 |
Research interests
Machine learning and scientific models
Effective integration of domain-specific prior knowledge / inductive bias (e.g., mathematical models of phenomena, simulators, logical rules, and side information) into statistical machine learning. For example, we developed a deep dynamics model with prior knowledge of stable invariant sets such as limit cycles. We formulated a method for learning dynamical systems with side information. We proposed a regularized learning method for properly learning a deep grey-box models, which are useful for robust and physically-interpretable generative modeling. We further discussed the learning methods of such models. Also interested in the simulation-based inference (SBI) as a related topic; for example, we studied a method for reliable SBI.
Data-driven analysis of dynamical systems
Analysis of dynamical systems based on data-driven methods, such as dynamic mode decomposition (DMD) and its variants. We proposed, e.g., Bayesian DMD, DMD for random dynamics, DMD with neural net observables, nonnegative DMD, time-varying DMD, and discriminant DMD for labeled time-series. We introduced the use of multiple kernel learning for diffusion-map-based Koopman analysis.