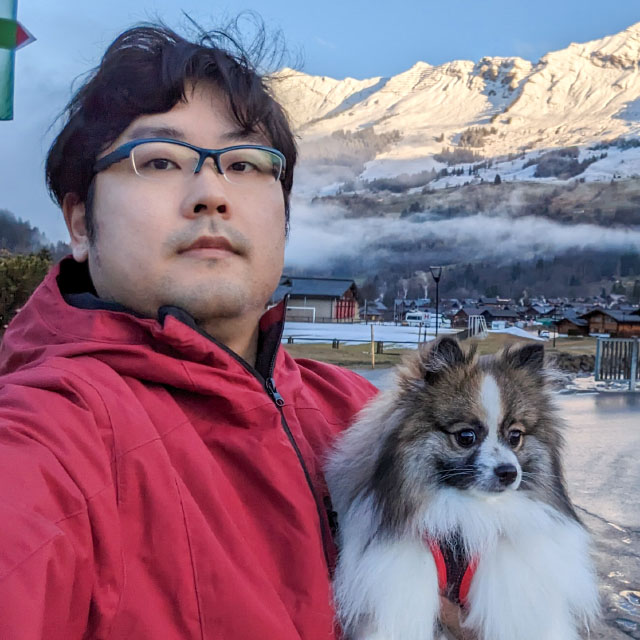
Naoya Takeishi is a researcher working at the University of Tokyo. He is interested in the effective integration of domain knowledge into statistical machine learning and also in data-driven analysis of dynamical systems, whose application ranges from scientific research to industrial machinery.
Contact
ntake[at]g.ecc.u-tokyo.ac.jp
I don’t observe the inbox of the old addresses (…@ailab.t.u-tokyo.ac.jp, …@hesge.ch, …@riken.jp).
Recent activities
2024-12-14 | I will be a panelist @ the workshop on Machine Learning and the Physical Sciences, NeurIPS 2024 |
---|---|
2024-10-22 | Invited talk on ML and scientific models @ The 2024 Fall Meeting of the Seismological Society of Japan [slides] |
2024-09-23 | I joined the Editorial Board of the journal Machine Learning: Science and Technology (MLST) |
2024-07-15 | Invited talk on neural nets and Koopman operator learning @ the workshop on Koopman Operators in Robotics, RSS 2024 [slides] |
2024-05-29 | Tutorial talk on ML and scientific models @ The 38th Annual Conference of the Japanese Society for Artificial Intelligence [slides] |
2023-08-03 | Invited talk on ML and scientific models @ The 46th Annual Meeting of the Japan Neuroscience Society |
2023-07-28 | Co-organized the workshop on Synergy of Scientific and ML Modeling @ ICML 2023 |
Research interests
Machine learning and scientific models
Effective integration of domain-specific prior knowledge / inductive bias (e.g., mathematical models of phenomena, simulators, logical rules, and side information) into statistical machine learning. For example, we developed a deep dynamics model with prior knowledge of stable invariant sets such as limit cycles. We formulated a method for learning dynamical systems with side information. We proposed a regularized learning method for properly learning a deep grey-box models, which are useful for robust and physically-interpretable generative modeling. We further discussed the learning methods of such models. Also interested in machine learning-based solution of forward problems (e.g., solving differential equations) and inverse problems (e.g., inference of simulation parameters). For example, we studied a method for reliable neural simulation-based inference.
Data-driven analysis of dynamical systems
Analysis of dynamical systems based on data-driven methods, such as dynamic mode decomposition (DMD) and its variants. We proposed, e.g., Bayesian DMD, DMD for random dynamics, DMD with neural net observables, nonnegative DMD, time-varying DMD, and discriminant DMD for labeled time-series. We introduced the use of multiple kernel learning for diffusion-map-based Koopman analysis.
Data-driven prognostics and health management
Application of anomaly detection techniques based on machine learning to engineering systems, such as artificial satellites, vehicles, and power plants, as well as methodology for interpretation of anomaly detection. For example, we investigated the use of Shapley values for interpreting semi-supervised anomaly detection.